How E-commerce Companies Can Use Data Analytics to Drive Growth (2025 Guide)
In the fast-paced world of digital commerce, e-commerce businesses can no longer rely on intuition or anecdotal evidence to make decisions. Data analytics has become the engine of sustainable growth, helping retailers understand customer behavior, optimize operations, and make proactive strategic decisions.
This article explores how e-commerce companies—from startups to enterprise brands—can leverage data analytics in e-commerce to improve efficiency, elevate customer experience, and outperform the competition.
Why Data Analytics is a Game-Changer for E-commerce
Modern e-commerce is defined not just by product availability or website design, but by the ability to harness insights in real time. Here’s why data analytics in e-commerce matters more than ever:
- Understand customer behavior in detail
- Optimize pricing and promotions based on demand
- Forecast inventory with high precision
- Personalize shopping experiences to increase conversions
- Monitor marketing ROI with actionable KPIs
- Reduce cart abandonment by identifying friction points in the journey
In short, data bridges the gap between potential and performance.
Key Applications of Data Analytics in E-commerce
1. Customer Insights and Personalization
By analyzing browsing behavior, purchase history, and interaction patterns, businesses can tailor user experiences, from homepage content to product recommendations. Modern data analytics platforms enable sophisticated customer segmentation and real-time personalization that was once only available to tech giants.
Benefits:
- Higher customer satisfaction
- Better repeat purchase rates
- Increased average order value
- Shorter conversion cycles
Use Case: Amazon and Netflix are prime examples of personalization powered by data. E-commerce businesses can use similar AI-driven techniques on a smaller scale.
2. Dynamic Pricing Optimization
With real-time analytics, businesses can adjust prices based on:
- Supply and demand
- Competitor pricing
- Customer segment value
Benefits:
- Maximized revenue without compromising customer trust
- Flexibility during seasonal events or flash sales
Pro Tip: Test price elasticity by running A/B pricing tests using historical and real-time data.
3. Inventory & Supply Chain Management
Predictive models help forecast demand patterns, reduce overstock or stockouts, and improve warehouse efficiency. This leads to better planning, less waste, and faster fulfillment. Advanced supply chain visualization tools provide real-time visibility into inventory movement and help identify bottlenecks before they impact customer experience.
Use Case: Apparel retailers can use weather + location data to predict which SKUs will spike in a region, optimizing stock movement.
4. Marketing Efficiency and Segmentation
Tracking KPIs like click-through rates, cost-per-acquisition, and return on ad spend allows for continuous campaign optimization. Following the latest data analytics trends, businesses are increasingly using predictive analytics and machine learning to identify high-value customer segments and optimize marketing spend.
Benefits:
- Higher ROAS (Return on Ad Spend)
- Lower customer acquisition cost
- More engaging campaigns that feel personalized
5. Customer Service Intelligence
Sentiment analysis on reviews and support tickets highlights recurring problems. Predictive tools can identify likely issues before they arise, helping teams proactively reduce friction. This approach mirrors strategies used in data analytics in banking industry, where predictive models help prevent customer churn and identify service opportunities.
Application: AI-powered chatbots trained with historical customer interaction data can automate and personalize support at scale.
6. Cart Abandonment Analysis
Analytics tools can identify exactly where users drop off in the checkout process. Is it at shipping cost? Payment options? Delay in page load?
Insight: By testing variables and analyzing behavioral patterns, companies can reclaim lost revenue by improving UX at specific friction points.
Establishing a Data-Driven Culture
1. Executive Alignment
Leadership must advocate for analytics-driven decision-making and invest in tools and talent.
2. Cross-Functional Collaboration
Marketing, operations, product, and IT teams must work together to eliminate silos and unlock shared insights.
3. Data-Literate Teams
Train employees to interpret and act on insights, not just generate reports. Empower everyone from merchandisers to marketers to leverage dashboards.
4. The Right Tools
Adopt cloud-based analytics platforms, customer data platforms (CDPs), and real-time dashboards that match your business scale. A comprehensive ecommerce software solution should integrate seamlessly with your existing analytics infrastructure to provide unified insights across all touchpoints.
5. KPI Ownership
Set clear ownership for key metrics across departments. Align on definitions and reporting frequency to ensure consistency.
Common Challenges to Watch For
- Poor Data Quality: Inaccurate or fragmented data leads to poor insights. Implement strong data governance.
- Integration Complexity: Legacy systems and disconnected tools can hinder unified analytics. Partnering with experts in software development for startups can solve these issues.
- Talent Gaps: Skilled data analysts and engineers are scarce. Consider staff augmentation to fill gaps quickly.
- Privacy & Compliance: Ensure GDPR/CCPA compliance and data protection by design.
- Siloed Reporting: Ensure that insights are shared across departments. Centralized dashboards promote shared accountability.
Measuring Success: What to Track
Analytics success isn’t just about dashboards. Look for measurable business outcomes:
- Revenue growth from personalized campaigns
- Reduced operational costs through automation
- Higher customer retention and lifetime value
- Better marketing ROI
- Faster decision-making with fewer errors
- Reduction in cart abandonment rates
- Improved fulfillment and inventory turnover
Savvycom: Your Data-Driven Development Partner
At Savvycom, we help e-commerce companies harness the power of data with tailored ecommerce software solutions that integrate analytics seamlessly into digital platforms. From building robust retail software to implementing machine learning models, we ensure your business is set up for long-term success.
With over 700 engineers and deep experience in AI, cloud, and e-commerce systems, Savvycom combines technical excellence with business insight.
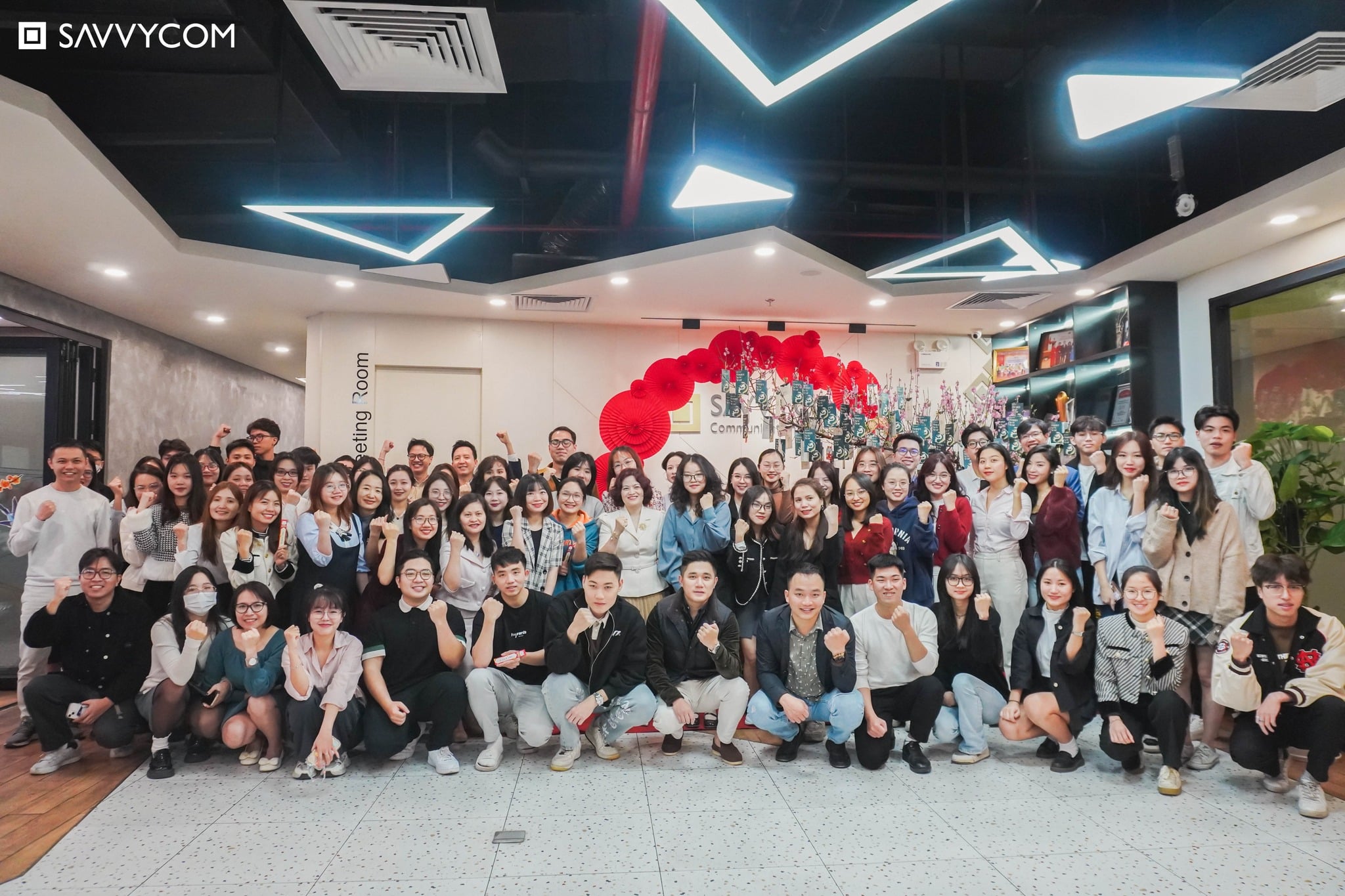
Savvycom’s proud to be the leading software development partner in Vietnam
Need help transforming your e-commerce platform into a data-powered growth engine?
Get in touch with our experts today.
FAQs
1. What is the most important use of data analytics in e-commerce?
Personalization. It directly impacts conversions, retention, and customer satisfaction.
2. Can small e-commerce businesses benefit from analytics?
Absolutely. Even basic tracking (e.g., Google Analytics, heatmaps) can offer powerful insights for improvement.
3. How do I know if my data strategy is working?
Monitor revenue lift, campaign ROI, retention rates, and decision speed. If metrics improve, your analytics are paying off.
4. What tools are best for e-commerce analytics?
Google Analytics 4, Mixpanel, Looker, Tableau, and AI-integrated platforms like CDPs are widely used.
5. Can Savvycom help integrate analytics into my e-commerce website?
Yes. We offer end-to-end solutions from data infrastructure to machine learning integration.
6. How is “Data Analytics in E-commerce” different from general analytics?
It focuses specifically on customer behavior, purchase trends, inventory cycles, and conversion optimization tailored to online commerce workflows.
7. Why is Data Analytics in E-commerce critical in 2025?
Because customer expectations, competition, and technology are evolving rapidly. Companies that act on data win. Those that don’t fall behind.