6 Stages of Data Processing: Transforming Raw Data into Strategic Insights
In an age where data is the new oil, understanding the stages of data processing is vital for businesses seeking to harness the power of information. This article will explore these stages in detail, emphasizing their significance and implications for various industries. By critically examining the processes involved, technologies available, and the future of data processing, we can gain deeper insights into how organizations can leverage data effectively.
As a leader in software outsourcing in Vietnam, Savvycom offers expertise in data processing technologies, helping businesses navigate the complexities of transforming data into valuable resources. This article delves into the key stages of data processing, the tools involved, and their applications, providing critical insights into how organizations can harness the power of data to drive growth and innovation.
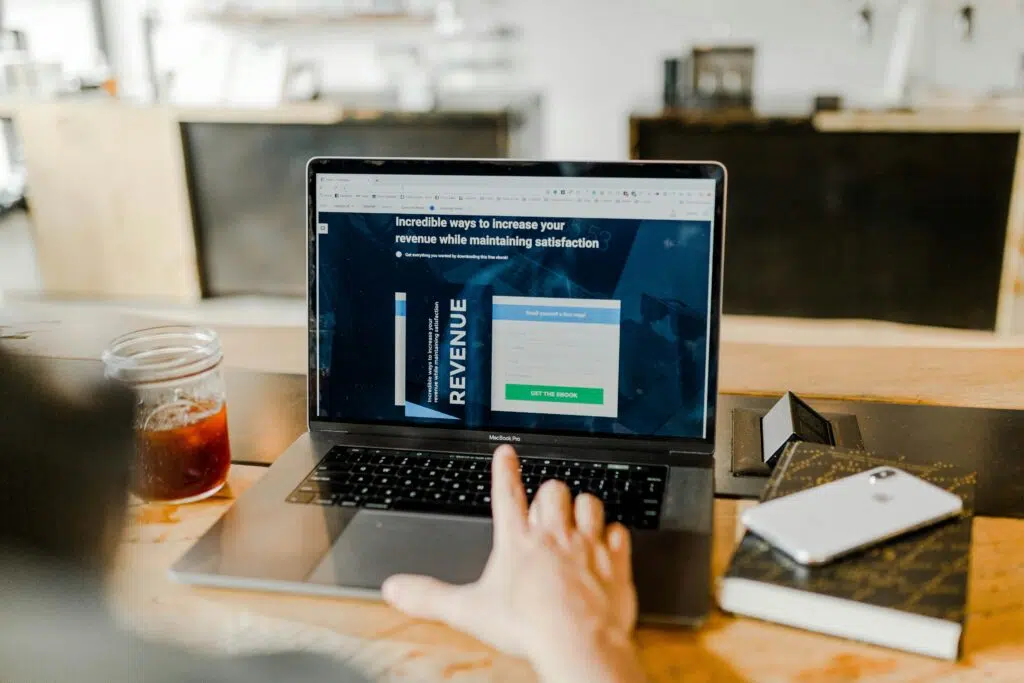
Explore 6 stages of data processing
What Are the Stages of Data Processing?
The stages of data processing encompass a systematic approach to handling data, transforming it from raw inputs to valuable insights. The typical stages can be categorized as follows:
1. Data Collection
Data collection is the foundational stage where information is gathered from various sources. This could include surveys, transactions, social media, sensors, and more. In today’s IoT Digital Transformation world, the volume of data being generated is staggering. According to Statista, the global data volume is expected to reach 175 zettabytes by 2025.
IIn this stage, it’s essential to ensure that the data collected is relevant and of high quality. Poor data quality assurance can have downstream effects, skewing results and leading to misguided conclusions.
2. Data Preparation
Once data is collected, it enters the data preparation stage, which involves cleaning, transforming, and organizing the data to ensure it is ready for analysis. This stage often includes removing duplicates, correcting errors, and addressing missing values.
This is arguably one of the most time-consuming stages of data processing. According to a study published in the Harvard Business Review, data scientists spend nearly 80% of their time on data preparation rather than analysis. The implications of this inefficiency highlight the need for robust data preparation tools and methodologies. Investing in quality data preparation solutions can drastically improve the overall efficiency of the data processing pipeline.
3. Data Processing
In the data processing stage, the prepared data undergoes various transformations to convert it into a structured format suitable for analysis. This could involve statistical analysis, aggregation, and application of algorithms to mine for insights.
Tools like ETL (Extract, Transform, Load) frameworks play a significant role in this stage. According to a report by Allied Market Research, the global ETL market is projected to reach $11.54 billion by 2026, growing at a CAGR of 8.9%. This growth reflects the increasing need for effective data processing solutions as organizations strive to make data-driven decisions. Additionally, leveraging data analytics trends can further enhance how businesses utilize processed data.
4. Data Analysis
Once the data is processed, it enters the data analysis stage, where analytics tools are applied to uncover trends, patterns, and insights. Advanced analytics methods, including predictive analytics and machine learning, can significantly enhance the depth of insights derived from the data.
As organizations increasingly adopt AI and machine learning, the need for skilled data analysts will become paramount, further emphasizing the critical nature of this stage.
5. Data Visualization and Interpretation
The final stage of data processing is data visualization, where the analyzed data is presented in an easily interpretable format. This includes charts, graphs, and dashboards that help stakeholders make informed decisions based on the insights provided.
Effective data visualization is crucial, this stage is where the insights come to life and drive strategic business decisions.
Key Technologies Driving Data Processing
The effectiveness of the stages of data processing is heavily influenced by the technologies employed in each phase. Here are some critical technologies shaping the future of data processing:
ETL Tools
ETL tools like Apache Nifi and Talend are crucial in the data processing landscape. They streamline the extraction, transformation, and loading of data, ensuring that the right data is in the right place at the right time.
Big Data Frameworks
Big data frameworks such as Apache Hadoop and Apache Spark facilitate the handling of vast datasets, enabling organizations to process and analyze data quickly. These frameworks support distributed computing, making it easier to run complex data processing tasks.
Data Warehousing Solutions
Data warehousing solutions, such as Snowflake and Amazon Redshift, provide organizations with scalable storage options that enable efficient data management and analysis. These platforms are essential for supporting complex queries and analytics.
Machine Learning and AI
The integration of machine learning and AI into data processing allows for advanced analytics capabilities, such as predictive modeling and automated insights generation. The rise of AI-driven analytics tools can significantly enhance the depth and speed of insights derived from data.
Cloud Computing
The shift toward cloud computing for business has revolutionized data processing. Cloud solutions provide scalability and flexibility, enabling organizations to store and analyze large datasets without substantial upfront investment. According to Harvard Business Review, by 2025, 85% of organizations will be cloud-first, underscoring the growing reliance on cloud-based data processing solutions.
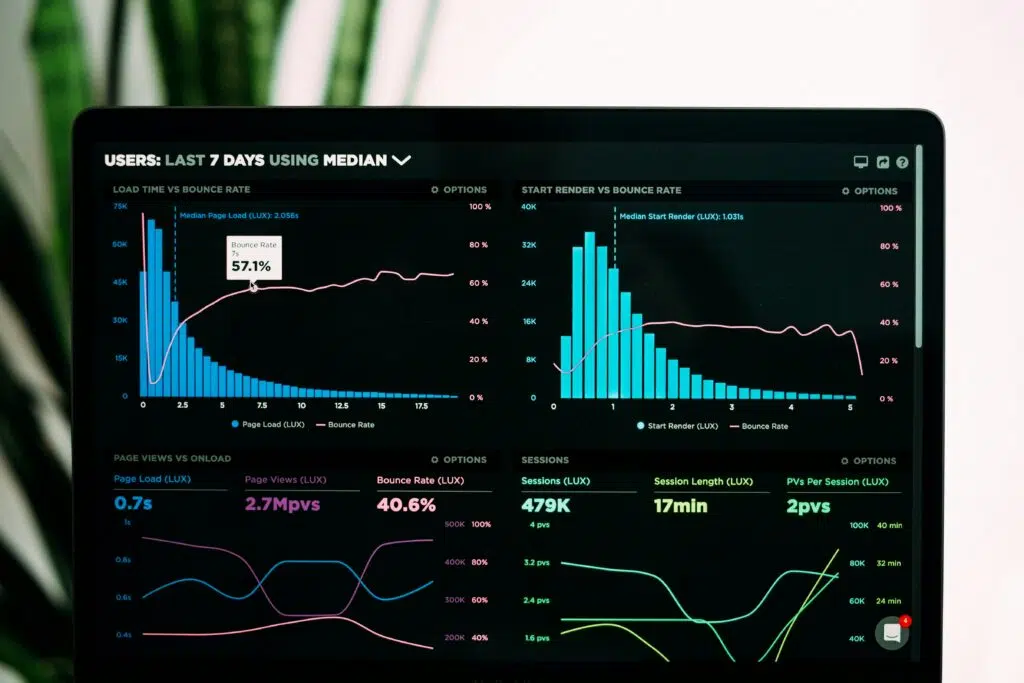
Leverage key technologies driving data processing
Applications of Data Processing Across Industries
Data processing plays a crucial role across various industries, leading to improved operations and decision-making. Here’s how different sectors are leveraging the stages of data processing:
Healthcare
In healthcare, data processing is essential for patient management, predictive analytics, and personalized medicine. For example, by analyzing patient data, healthcare providers can predict disease outbreaks and recommend tailored treatment plans. The healthcare analytics market is expected to reach $50 billion by 2024, according to Markets and Markets. Healthcare analytics companies are instrumental in transforming healthcare data into actionable insights for better patient outcomes.
Finance
The finance sector utilizes data processing for risk management, fraud detection, and customer segmentation. According to a report by Accenture, organizations using data analytics in finance can improve profitability by up to 20%.
Retail
In the retail sector, retailers leverage data processing for inventory management, customer behavior analysis, and personalized marketing. Additionally, implementing RPA in retail can further streamline operations by automating repetitive tasks and improving data accuracy.
Manufacturing
In manufacturing, data processing is critical for optimizing production processes and predictive maintenance. A study by PwC estimates that predictive maintenance can reduce maintenance costs by 25-30%, significantly enhancing operational efficiency.
The Future of Data Processing
As we look toward the future, several trends are likely to shape the stages of data processing:
Artificial Intelligence and Machine Learning
AI and machine learning will continue to enhance data processing capabilities, enabling organizations to derive deeper insights from their data. According to a report by PwC, AI could add $15.7 trillion to the global economy by 2030, illustrating its transformative potential.
Edge Computing
With the rise of IoT devices, edge computing is becoming increasingly relevant. By processing data closer to the source, organizations can reduce latency and bandwidth usage, leading to faster decision-making.
Increased Data Privacy Regulations
As data processing practices evolve, so do regulations surrounding data privacy. Compliance with laws like GDPR and CCPA is becoming critical, driving the need for secure data processing frameworks that prioritize user privacy.
For a deeper understanding of the transformative potential of data, we encourage you to watch this insightful video:
Frequently Asked Questions (FAQs)
How does the choice of technology impact the efficiency of data processing stages?
The technology used in the stages of data processing greatly impacts efficiency. For instance, advanced ETL tools like Talend or Apache Nifi can automate data cleaning and transformation, significantly reducing time spent on these tasks. Similarly, utilizing big data frameworks such as Apache Spark enables organizations to process vast datasets quickly. A study from Forbes suggests that companies leveraging cloud-based solutions for data processing report a 20-30% increase in efficiency. Therefore, selecting the right technology stack is crucial for optimizing data processing workflows.
What are the common challenges organizations face during data processing?
Organizations often encounter several challenges in the stages of data processing. These include:
- Data Quality Issues: Inaccurate or incomplete data can lead to misleading insights. Research by Gartner indicates that poor data quality costs organizations an average of $15 million annually.
- Integration Problems: Integrating disparate data sources can be complex and time-consuming, especially in organizations with legacy systems.
- Scalability: As data volume grows, many organizations struggle to scale their data processing solutions effectively. A report by McKinsey highlights that 70% of companies feel unprepared for the data challenges they face today.
- Regulatory Compliance: Ensuring compliance with data privacy laws, such as GDPR, can complicate data processing efforts, requiring additional resources and oversight.
How can businesses measure the effectiveness of their data processing stages?
To measure the effectiveness of the stages of data processing, businesses can use several key performance indicators (KPIs):
- Data Quality Metrics: Track metrics such as accuracy, completeness, and consistency of the data.
- Processing Time: Measure the time taken at each stage to identify bottlenecks and inefficiencies.
- User Satisfaction: Collect feedback from end-users on the insights generated and their usefulness in decision-making.
- Return on Investment (ROI): Calculate the ROI from data-driven initiatives to assess the financial impact of improved data processing.
What best practices should organizations adopt for effective data processing?
Organizations can adopt several best practices to enhance the stages of data processing:
- Invest in Data Governance: Implementing robust data governance policies ensures data quality, security, and compliance.
- Automate Where Possible: Utilizing automation tools for data collection, cleaning, and transformation can save time and reduce errors.
- Focus on Training: Providing training for employees on data handling and analytics tools can enhance overall effectiveness.
- Regularly Review Processes: Continuous assessment and optimization of data processing workflows help organizations adapt to changing business needs and technologies.
Final Thoughts
The stages of data processing form the backbone of effective data management. Understanding these stages enables organizations to harness data as a strategic asset, driving innovation and efficiency. As technology evolves, the integration of AI, cloud computing, and robust data governance frameworks will continue to shape the landscape of data processing.
For organizations seeking to enhance their data processing capabilities, partnering with a reliable software development company can provide the expertise needed to implement effective data solutions. At Savvycom, we specialize in data processing and analytics, helping businesses harness the power of their data to achieve meaningful results. Contact us today to learn how we can assist you!