Data Quality Assurance: Key Metrics to Measure Success
In an increasingly data-driven world, the integrity and quality of data have become paramount for organizations striving to make informed decisions. Data Quality Assurance (DQA) encompasses a series of processes designed to ensure that the data used within an organization is accurate, consistent, and reliable. According to a study by Gartner, organizations can lose up to $12.9 million annually due to poor data quality. This staggering figure underscores the necessity for businesses, especially those relying on technology solutions provided by a software development company, to prioritize data quality assurance as a fundamental component of their operations.
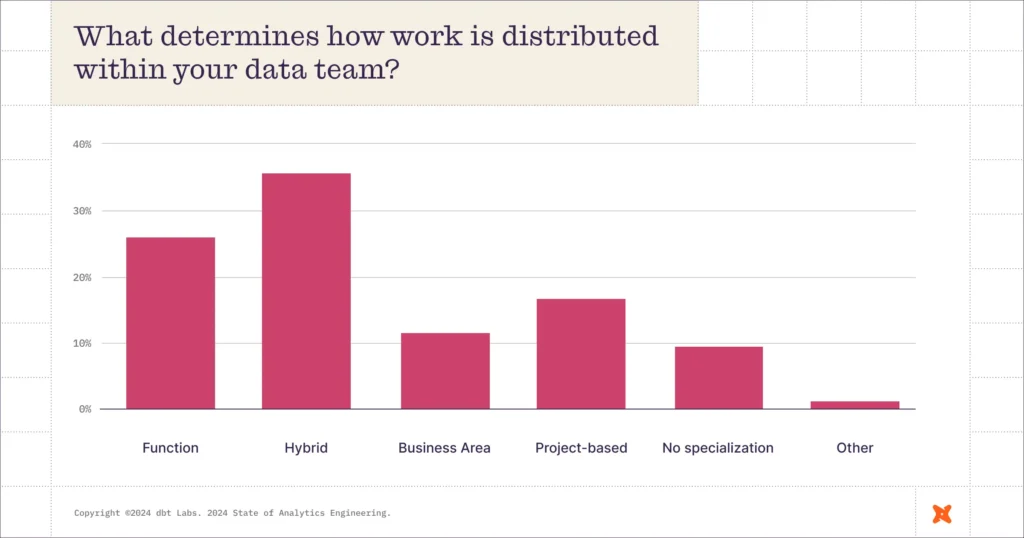
Image source: Getdbt
Data quality assurance refers to the systematic processes and methodologies employed to verify, validate, and maintain the integrity of data throughout its lifecycle. This framework aims to detect and rectify errors, inconsistencies, and inaccuracies in data, thereby ensuring that the information used for decision-making is reliable.
The importance of DQA cannot be overstated. Reliable data is essential for organizations to thrive in competitive markets. It influences decision-making processes, operational efficiency, and customer satisfaction. Moreover, DQA plays a crucial role in compliance with industry regulations, helping businesses avoid legal repercussions associated with inaccurate or misleading data.
A 2022 report from IBM indicated that companies actively managing data quality are three times more likely to experience significant business benefits compared to those that neglect this aspect. This statistic highlights the direct correlation between robust data quality practices and business success.
What are Examples of Data Quality Assurance?
Data quality assurance encompasses various practices designed to enhance the quality of data across different domains. Some common examples of DQA include:
-
Data Validation Techniques: This involves implementing rules and criteria to ensure that data meets specific standards. For example, a financial institution may validate that all transaction amounts are numeric and fall within an acceptable range.
-
Data Cleansing Processes: This practice involves identifying and correcting errors or inconsistencies in datasets. For instance, a healthcare provider may cleanse patient records to remove duplicates or correct misspellings.
-
Regular Audits and Monitoring: Conducting periodic audits of data can help organizations identify areas for improvement. Continuous monitoring ensures that any anomalies are detected and addressed promptly.
According to a survey by IBM, 94% of executives reported that poor data quality negatively impacts their business, demonstrating the critical need for DQA practices in organizations.
The Value of Reliable Data to the Business
Reliable data forms the backbone of successful business operations, enabling organizations to make informed decisions and drive growth. The impact of reliable data extends to various aspects of business performance:
-
Enhanced Decision-Making Processes: Access to accurate and timely data allows executives to make well-informed decisions, leading to improved business outcomes. A McKinsey study revealed that data-driven organizations are 23 times more likely to acquire customers, 6 times more likely to retain them, and 19 times more likely to be profitable.
-
Improved Customer Satisfaction and Trust: Organizations that prioritize data quality build trust with their customers. Reliable data allows for personalized marketing efforts and better customer service, resulting in higher satisfaction rates.
-
Increased Operational Efficiency and Cost Savings: By maintaining high data quality, organizations can streamline processes and reduce errors. This leads to significant cost savings, as resources are allocated more effectively.
Research indicates that companies with high data quality can increase productivity by up to 25%, highlighting the tangible benefits of reliable data.
Data Quality Assurance Use Cases
Different industries face unique challenges regarding data quality, making DQA crucial for success. Here are several use cases that illustrate the importance of DQA in various sectors:
Financial Services
In the financial services sector and financial software development, data accuracy is paramount. Institutions rely on accurate data for transactions, financial risk management, and regulatory compliance. A single error in transaction data can lead to significant financial losses and regulatory penalties. Therefore, financial organizations invest heavily in DQA practices to ensure the integrity of their data.
Healthcare
Acrodding to top healthcare analytics companies, reliable data is essential for patient safety and regulatory compliance. Accurate patient records enable healthcare providers to deliver effective treatment and ensure compliance with regulations. A study from the American Journal of Managed Care found that organizations prioritizing data quality see a 20% improvement in patient outcomes. This statistic emphasizes the critical role of DQA in enhancing healthcare delivery, specially when you are having outsourcing services in healthcare.
Retail
In the retail industry, data quality impacts inventory management, customer insights, and personalized marketing efforts. Reliable data enables retailers to manage stock levels effectively, understand customer preferences, and tailor marketing campaigns accordingly.
Have a Project Idea in Mind?
Get in touch with Savvycom’s experts for a free consultation. We’ll help you decide on next steps, explain how the development process is organized, and provide you with a free project estimate.
Data Quality Assurance Best Practices
Implementing best practices is essential for organizations aiming to enhance their data quality assurance initiatives. Here are some effective best practices:
-
Define Clear Data Quality Metrics and Objectives: Establishing specific metrics allows organizations to measure data quality effectively. This may include metrics such as accuracy, completeness, consistency, and timeliness.
-
Invest in Data Quality Tools and Technologies: Organizations should invest in specialized tools that facilitate data validation, cleansing, and monitoring. Tools such as data profiling software can help identify data quality issues before they escalate.
-
Foster a Data-Driven Culture: Encouraging a culture that prioritizes data quality at all levels of the organization is crucial. Employees should understand the importance of maintaining high data quality standards.
-
Conduct Regular Training for Staff: Training employees on data quality practices ensures they are equipped with the knowledge and skills needed to uphold data quality standards.
According to a report by PwC, organizations that adopt best practices for data quality can enhance their decision-making speed by 30%. This statistic reinforces the value of implementing effective DQA practices.
Getting Started with Data Quality Assurance in 9 Steps
Initiating a data quality assurance program can be simplified into a series of actionable steps. Here’s a nine-step guide to get started:
-
Assess Current Data Quality Status: Conduct a thorough assessment of the current state of your data. Identify existing issues and areas for improvement.
-
Define Data Quality Objectives: Establish clear objectives for what you want to achieve with your DQA program. This could include specific accuracy or completeness goals.
-
Identify Key Data Sources: Determine which data sources are critical to your operations and prioritize them for DQA efforts.
-
Establish Metrics for Measuring Data Quality: Define the key metrics that will be used to measure data quality, such as error rates or data completeness percentages.
-
Implement Data Quality Tools and Technologies: Invest in tools that facilitate data quality management, including data profiling, cleansing, and monitoring solutions.
-
Train Staff on Data Quality Practices: Ensure that employees are trained in data quality standards and practices, empowering them to contribute to data quality initiatives.
-
Monitor and Measure Data Quality Regularly: Continuously monitor data quality using established metrics and adjust strategies as needed.
-
Address Identified Issues Promptly: Take immediate action to rectify any data quality issues that arise to prevent them from escalating.
-
Continuously Improve Data Quality Processes: Regularly review and refine data quality processes to ensure ongoing improvement.
A structured approach to DQA can lead to a 40% increase in data quality effectiveness, making it essential for organizations to follow these steps diligently.
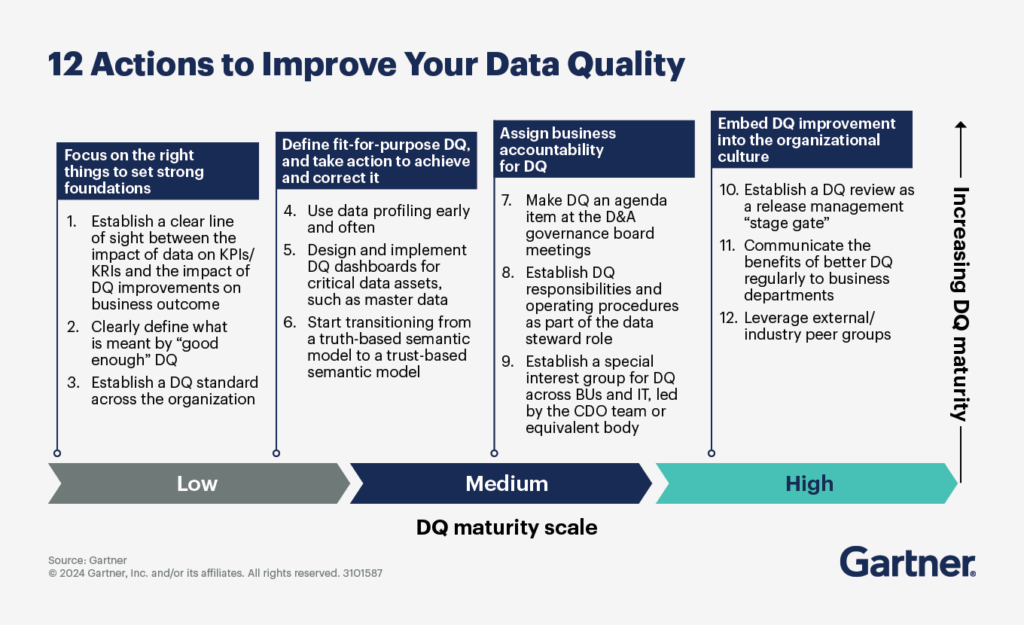
Image source: Gartner
How a Data Intelligence Platform Can Help You Support Data Quality
Leveraging a data intelligence platform can significantly enhance an organization’s data quality assurance efforts. These platforms offer a range of functionalities that facilitate data quality management:
-
Data Cleansing and Validation: Data intelligence platforms often include built-in data cleansing tools that automatically identify and rectify errors, ensuring that only high-quality data is utilized.
-
Continuous Monitoring: These platforms enable continuous monitoring of data quality, allowing organizations to detect anomalies and issues in real time.
-
Real-Time Insights: Data intelligence platforms provide real-time insights into data quality metrics, enabling organizations to make informed decisions promptly.
-
Automated Reporting Features: Automated reporting capabilities streamline the process of generating data quality reports, saving time and resources.
Research indicates that organizations using data intelligence platforms report a 30% reduction in data errors, demonstrating the effectiveness of these tools in supporting data quality assurance efforts.
Conclusion
In conclusion, data quality assurance is an essential aspect of modern business operations. Organizations that prioritize data quality reap significant benefits, including improved decision-making, enhanced customer satisfaction, and increased operational efficiency. By implementing best practices, following a structured approach, and leveraging data intelligence platforms, businesses can ensure the reliability of their data and drive success in an increasingly competitive landscape.
As the saying goes, “garbage in, garbage out.” Investing in data quality assurance is not just an option; it’s a necessity for organizations looking to thrive in today’s data-centric world. By taking proactive steps to enhance data quality, businesses can unlock the full potential of their data and set themselves up for long-term success.
Savvycom, a leading software development company, understands the critical role of data quality assurance in ensuring the success of modern businesses. By offering cutting-edge solutions in data management and intelligence, Savvycom helps organizations across industries establish effective governance frameworks, streamline with full stages of data processing, and enhance the overall reliability of their data. Savvycom enables companies to improve decision-making, elevate customer experiences, and optimize operational efficiencies. By partnering with Savvycom, businesses can harness the power of high-quality data to gain a competitive edge and drive sustained growth in today’s data-driven world.
Tech Consulting, End-to-End Product Development, Cloud & DevOps Service! Since 2009, Savvycom has been harnessing digital technologies for the benefit of businesses, mid and large enterprises, and startups across the variety of industries. We can help you to build high-quality software solutions and products as well as deliver a wide range of related professional services.
Savvycom is right where you need. Contact us now for further consultation:
- Phone: +84 24 3202 9222
- Hotline: +1 408 663 8600 (US); +612 8006 1349 (AUS); +84 32 675 2886 (VN)
- Email: [email protected]