Statistical Process Control in Manufacturing: Maximizing Quality and Efficiency in the Digital Age
In today’s hyper-competitive industrial landscape, statistical process control in manufacturing has emerged as an essential strategy for optimizing production quality, reducing waste, and enhancing overall operational efficiency. Manufacturers across BFSI, healthcare, retail, and manufacturing sectors are increasingly relying on data-driven methods to monitor, control, and improve production processes. By integrating real-time data and advanced analytics, statistical process control transforms raw production data into actionable insights that drive continuous improvement.
This article explores the critical importance of statistical process control in manufacturing, delves into its key components, examines real-world case studies, and offers practical strategies for implementation – all while providing deeper insights and critical analysis to help you optimize your production processes. Moreover, partnering with a leading software development company ensures access to expert guidance and custom solutions tailored to your specific manufacturing challenges.
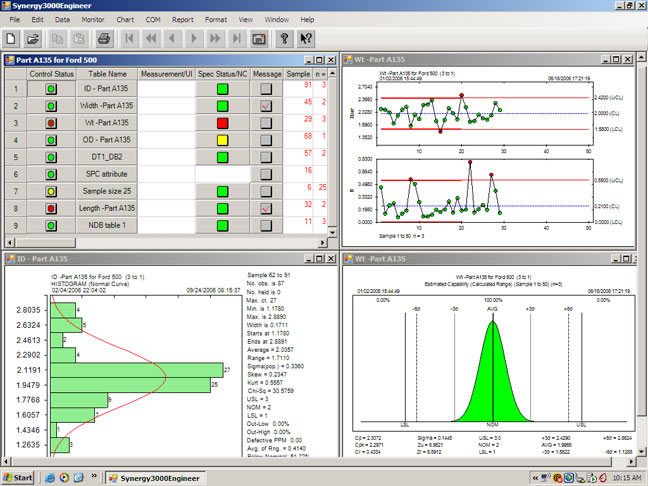
Source: Latest Quality
Understanding Statistical Process Control in Manufacturing
Statistical process control in manufacturing is a systematic method used to monitor and control production processes using statistical techniques. At its core, SPC is designed to reduce variability and defects by identifying process deviations early. By applying statistical methods to quality data, manufacturers can predict potential issues, make informed adjustments, and ensure that production processes remain stable and efficient.
Unlike traditional quality control methods that rely on periodic inspections, SPC continuously measures process performance using control charts and other statistical tools. This proactive approach allows for real-time decision-making and minimizes the likelihood of defective products reaching customers. According to International Journal of Production Research, companies that implement SPC effectively can reduce process variability by up to 70%, thereby significantly improving product quality and reducing overall production costs.
Core Components of Statistical Process Control in Manufacturing
1. Data Collection and Integration
A robust SPC system begins with the comprehensive collection and integration of data. In manufacturing, data is gathered from a variety of sources, sensors on production machines, manual entries from operators, ERP systems, and even IoT devices. These data points, when integrated, create a unified view of the production process.
Efficient data integration ensures that information flows seamlessly between different production stages. Modern ETL (Extract, Transform, Load) tools and APIs facilitate the consolidation of disparate data sources, providing a “single source of truth” for analysis. Improved data integration can lead to a 40% reduction in process errors.
2. Control Charts and Real-Time Monitoring
Control charts are the cornerstone of statistical process control in manufacturing. These visual tools display process data over time and include control limits that help identify when a process is deviating from its expected performance. By continuously monitoring these charts, operators can quickly detect anomalies and implement corrective actions before defects occur.
3. Advanced Analytics and Predictive Modeling
The integration of predictive analytics into SPC systems is revolutionizing how manufacturers forecast issues. Machine learning algorithms can analyze historical and real-time data to predict future deviations or equipment failures. These predictive models enable proactive maintenance, ensuring that potential problems are addressed before they result in costly downtime.
4. Customization and Scalability
Every manufacturing process is unique, and a one-size-fits-all approach to SPC is rarely effective. Customizable SPC solutions allow manufacturers to tailor control charts, reporting tools, and alert mechanisms to match their specific production processes and quality requirements. Scalability is equally important, an effective SPC system must grow with the business and adapt to changes in production volume and complexity.
Looking For a Trusted Tech Partner?
We’ll help you decide on next steps, explain how the development process is organized, and provide you with a free project estimate.
Why Statistical Process Control in Manufacturing Matters
Enhancing Quality Assurance
The primary goal of statistical process control in manufacturing is to enhance quality assurance. By constantly monitoring production processes and promptly addressing deviations, manufacturers can ensure that only products meeting strict quality standards are delivered to customers. This not only improves customer satisfaction but also reduces warranty claims and recalls.
Reducing Operational Costs
Implementing SPC leads to significant cost savings by reducing waste, rework, and downtime. Automated monitoring and real-time analytics allow manufacturers to pinpoint inefficiencies and optimize resource allocation, thereby cutting operational costs by 20-30%. In industries where every minute of downtime can result in substantial financial losses, these savings are critical for maintaining profitability.
Driving Continuous Improvement
Statistical process control is inherently focused on continuous improvement. The data collected through SPC systems provides a basis for iterative process enhancements. Manufacturers can identify trends over time, understand the root causes of defects, and implement corrective actions that lead to ongoing improvements. This culture of continuous improvement is essential for staying competitive in a rapidly evolving market.
Supporting Data-Driven Decision Making
In the digital age, data is the most valuable asset. Statistical process control in manufacturing provides real-time insights that enable managers to make informed, data-driven decisions. This proactive approach allows for quick adjustments to production processes, ensuring optimal performance even in the face of changing market conditions. A study by McKinsey found that data-driven decision-making can boost productivity by up to 20%, underscoring the strategic importance of SPC.
Ensuring Regulatory Compliance
For industries such as pharmaceuticals, food production, and aerospace, regulatory compliance is non-negotiable. SPC systems help manufacturers maintain stringent quality standards and provide detailed audit trails that are essential for regulatory inspections. By ensuring that production processes are consistent and well-documented, statistical process control helps reduce the risk of non-compliance and associated penalties.
Case Studies: Real-World Impact of Statistical Process Control
Case Study 1: Automotive Manufacturing Excellence
Background:
A major automotive manufacturer struggled with high variability in its welding process, leading to increased defect rates and rework costs.
Solution:
The company implemented a comprehensive SPC system that integrated data from IoT sensors on welding machines. Real-time control charts were used to monitor the quality of welds, while predictive analytics forecasted potential failures.
Results:
- 30% reduction in welding defects.
- 25% decrease in rework costs.
- 20% improvement in overall production efficiency.
This case clearly demonstrates how statistical process control in manufacturing can drive significant quality improvements and cost savings by enabling proactive monitoring and maintenance.
Case Study 2: Electronics Production Optimization
Background:
An electronics manufacturer faced issues with inconsistent product quality and high scrap rates on its assembly line, resulting in substantial financial losses.
Solution:
By deploying an SPC system, the manufacturer was able to consolidate data from various stages of production into a unified dashboard. Advanced analytics were used to set dynamic control limits, allowing operators to identify and correct process variations promptly.
Results:
- 25% improvement in production yield.
- 20% reduction in scrap rates.
- Enhanced consistency across production lines, leading to improved customer satisfaction.
This example underscores the transformative power of statistical process control in manufacturing in streamlining production processes and reducing waste, ultimately boosting profitability.
Case Study 3: Streamlining Quality Control in Food Processing
Background:
A leading food processing company experienced significant variability in product quality, resulting in high levels of waste and customer complaints.
Solution:
The company implemented an SPC system across its production lines to monitor critical parameters such as temperature, humidity, and mixing times. The system provided real-time alerts and detailed trend analyses, enabling rapid corrective actions.
Results:
- 20% reduction in material waste.
- 15% improvement in product consistency.
- 10% increase in customer satisfaction ratings.
This case illustrates how effective use of statistical process control in manufacturing can lead to substantial operational improvements in quality and efficiency, crucial for industries where product consistency is vital.
Future Trends in Statistical Process Control in Manufacturing
1. Integration of AI and Machine Learning
The future of statistical process control in manufacturing lies in the integration of AI and machine learning. These technologies will enable even more sophisticated predictive analytics, allowing manufacturers to anticipate process deviations and optimize control limits dynamically. Research by IBM suggests that AI-enhanced SPC can reduce downtime by an additional 15-20% compared to traditional methods.
2. Expansion of IoT Connectivity
With the proliferation of IoT devices, manufacturers will have access to even richer datasets. Enhanced sensor technology and connectivity will enable more precise real-time monitoring, allowing for finer control over production processes. As IoT integration becomes standard, the overall efficiency and accuracy of SPC systems are expected to improve significantly.
3. Adoption of Cloud-Based SPC Solutions
Cloud computing is transforming how data is stored and processed. Cloud-based SPC solutions offer scalability, flexibility, and cost-effectiveness, making them accessible for both large enterprises and smaller manufacturers. These solutions enable real-time data analysis and remote monitoring, ensuring that production processes remain efficient regardless of location.
4. Advanced Data Visualization and User Experience
Future SPC systems will benefit from advances in data visualization, providing more intuitive and interactive dashboards. Enhanced visualization tools will allow operators to drill down into specific metrics, identify trends quickly, and make informed decisions faster. This focus on user experience will drive greater adoption of SPC tools across the manufacturing floor.
5. Increased Focus on Cybersecurity and Compliance
As manufacturing systems become more interconnected, ensuring the security and integrity of production data is paramount. Future SPC solutions will incorporate advanced cybersecurity measures to protect sensitive data and ensure compliance with global standards such as GDPR, HIPAA, and PCI DSS. This emphasis on security will be critical for maintaining operational continuity and safeguarding intellectual property.
FAQs
How does SPC help reduce production costs?
By detecting process deviations in real time and enabling prompt corrective actions, SPC minimizes downtime, reduces scrap rates, and lowers the need for costly rework. Studies have shown that effective SPC implementation can reduce production costs by 20-30%.
What tools are commonly used for statistical process control?
Manufacturers utilize control charts, process capability analysis, and advanced analytics platforms such as Minitab and JMP to visualize and analyze production data. These tools help in identifying trends and establishing control limits for critical process parameters.
Can small manufacturers benefit from SPC?
Yes, small and medium-sized manufacturers can leverage cloud-based SPC solutions to gain real-time insights into their production processes, improve product quality, and reduce operational costs without significant upfront investments.
For additional insights, don’t miss this video:
Conclusion
In an era defined by digital transformation, statistical process control in manufacturing is an indispensable strategy for optimizing production quality and efficiency. By leveraging advanced data analytics, real-time monitoring, and predictive maintenance, manufacturers can significantly reduce variability, minimize defects, and achieve substantial cost savings. As competition intensifies and customer expectations rise, a robust SPC system provides the strategic insights necessary for continuous improvement and sustainable growth.
Investing in a well-designed SPC solution not only boosts operational efficiency but also drives data-driven decision-making, enabling manufacturers to stay agile and competitive in a rapidly evolving market. The future of manufacturing lies in harnessing the full potential of statistical process control, transforming raw production data into actionable insights that drive innovation.
Tech Consulting, End-to-End Product Development, Cloud & DevOps Service! Since 2009, Savvycom has been harnessing digital technologies for the benefit of businesses, mid and large enterprises, and startups across the variety of industries. We can help you to build high-quality software solutions and products as well as deliver a wide range of related professional services.
Savvycom is right where you need. Contact us now for further consultation:
- Phone: +84 24 3202 9222
- Hotline: +1 408 663 8600 (US); +612 8006 1349 (AUS); +84 32 675 2886 (VN)
- Email: [email protected]